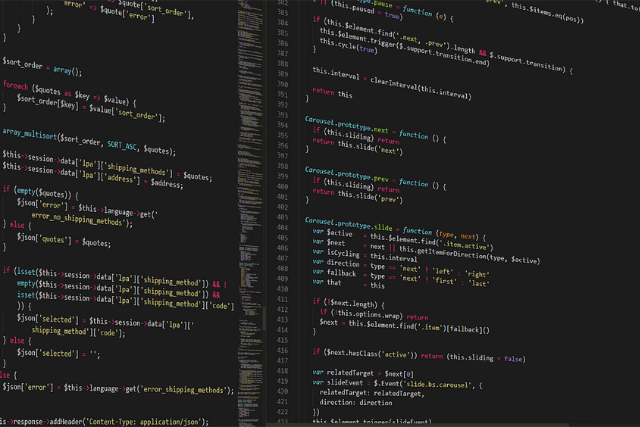
Artificial intelligence (AI) is at the vanguard in the fast-changing technological world, propelling innovation across sectors.
AI systems are deployed in all industries, from healthcare to finance. They can solve complex problems, enhance decision-making, and automate tasks. Statista said the global AI technologies market was valued at $200 billion in 2023. Furthermore, it is expected to grow multifold quickly to reach $1.8 trillion by 2030.
However, these systems' efficiency heavily depends on their capacity to learn and adapt over time. Continuous learning is thus an essential component of the AI pipeline, ensuring that models stay relevant, accurate, and efficient in changing contexts.
In this post, we will explore the significance and benefits of continuous learning in the AI pipeline.
The Dynamics of AI Models
AI models are fundamentally data-driven, particularly those leveraging machine learning (ML) and deep learning (DL) techniques. They discover patterns and generate predictions depending on the data they are trained with. However, the data landscapes in real-world applications are far from static.
They evolve due to various factors, such as changes in user behavior, market dynamics, regulatory updates, and emerging trends. Consequently, an AI model that performs well today might become obsolete tomorrow if it is not updated to reflect these changes.
Therefore, not training them with fresh data or using too much synthetic data can lead to model collapse. As defined by a TechTarget article, this phenomenon occurs when AI models, especially generative AI, do not get enough fresh human-curated data. This leads to the model forgetting the basic underlying data distribution, causing the AI to produce less diverse responses.
Continuous learning addresses this challenge by enabling AI models to be updated regularly with new data and insights. This process involves retraining models on fresh data, fine-tuning existing parameters, and redefining the model architecture to better capture new patterns. The goal is to maintain the model's performance and ensure it adapts to new information without significant degradation.
Advantages of Continuous Learning
Updating and refining AI models by incorporating new data and insights over time offers numerous benefits, such as:
Enhanced Accuracy and Relevance
One of the primary benefits of continuous learning is the sustained accuracy of AI models. As new data becomes available, models may be updated to represent the most recent trends and patterns. This continuous adaptation helps in maintaining high prediction accuracy and ensures that the AI system remains relevant and useful over time.
However, as an Oracle article mentions, some of the most significant challenges of training AI models are data-related. Insufficient or unclean quality data can lead to poor training and inaccurate outputs. Moreover, managing data can be extremely difficult and time-consuming.
Therefore, Dataloop emphasizes leveraging a robust AI development platform to help create an active learning pipeline. For instance, automated or pre-built pipeline templates can help curate and clean every piece of structured and unstructured data. This can prove useful in retrieval and filtering, thereby simplifying data selection.
Resilience to Concept Drift
In many applications, the underlying data distribution can shift gradually or suddenly, a phenomenon known as concept drift. For instance, consumer preferences can change due to seasonal trends, economic factors, or social influences. Continuous learning allows AI models to detect and adapt to concept drift, thereby maintaining their performance in the face of changing data distributions.
Incremental Learning
Continuous learning supports incremental learning, which involves updating models progressively as new data enters rather than retraining from scratch. This technique uses less processing resources and takes less time.
It also allows the AI system to incorporate new knowledge without forgetting previously learned information, a challenge known as catastrophic forgetting. Another solution has been found to mimic sleeplike phases to prevent this amnesia problem.
According to IEEE Spectrum, some researchers introduced these phases in spiking neural networks, and the results helped prevent catastrophic forgetting. Sadly, this procedure doesn't work with standard neural networks. However, the good part is that the researchers who conducted this experiment state that technological advancements will make it possible.
Scalability and Flexibility
As AI models are fed with continuous streams of data, they become more proficient at identifying patterns and making accurate predictions. This scalability is crucial for applications that process large datasets in real-time, such as financial markets, e-commerce, and autonomous systems.
Flexibility is another key benefit provided by continuous learning in AI pipelines. In a dynamic environment where data trends and user behaviors change frequently, AI models must adapt quickly to maintain relevance and accuracy.
Continuous learning frameworks allow models to be updated incrementally with new data, ensuring they remain aligned with current trends and emerging patterns. This flexibility reduces the risk of model obsolescence and enhances the system's ability to provide accurate, up-to-date insights.
Challenges and Considerations
While continuous learning has several advantages, it also poses obstacles that must be addressed. One of the most significant concerns is the possibility of overfitting, in which models become excessively tuned to current data and lose their generalizability.
As highlighted in a Springer Journal article, avoiding overfitting is crucial for high generalization and, thus, the AI model's success. Regular validation and cross-validation techniques are necessary to mitigate this risk.
Another problem is the computational expense of continual learning. Retraining models, particularly large-scale deep learning models, can be resource-costly. Cloud-based solutions and distributed computing can assist in controlling these expenses and scale the learning process more effectively.
Data management also poses a challenge. As data volumes grow, ensuring data quality and relevance becomes increasingly complex. Addressing this issue requires the implementation of strong data governance principles and the use of modern data management solutions.
Lastly, maintaining human oversight in the continuous learning process is challenging. While automation plays a significant role, human experts must interpret model outputs and make critical decisions.
Frequently Asked Questions
Why is the pipeline crucial in machine learning?
Pipelines are significant in machine learning because they guarantee systematic and efficient data processing across several stages. This organized method aids in preserving consistency, eliminating mistakes, and enabling scalability, making it easier to handle complicated operations.
What is continuous learning in artificial intelligence?
Continuous learning in AI is training AI models with fresh data and feedback to enhance their performance over time. This strategy enables AI systems to adapt to changing surroundings, learn from new data, and remain relevant in dynamic scenarios.
What function does data play in continuous artificial intelligence learning?
Data is vital in continuous AI learning since it is the foundation for model updates and upgrades. High-quality, diversified, and timely data enables the AI system to learn successfully from fresh instances and scenarios.
What are some problems related to integrating continuous learning in AI pipelines?
Implementing continuous learning in AI pipelines presents numerous issues. These include managing the volume and diversity of incoming data, guaranteeing data quality and consistency, and dealing with privacy and security issues.
To summarize, continuous learning is an indispensable component of the AI pipeline. It enables AI systems to remain accurate, relevant, and resilient despite evolving data landscapes. Organizations may maximize the promise of AI by embracing continuous learning, which drives innovation and maintains a competitive advantage.
(0) comments
We welcome your comments
Log In
Post a comment as Guest
Keep it Clean. Please avoid obscene, vulgar, lewd, racist or sexually-oriented language.
PLEASE TURN OFF YOUR CAPS LOCK.
Don't Threaten. Threats of harming another person will not be tolerated.
Be Truthful. Don't knowingly lie about anyone or anything.
Be Nice. No racism, sexism or any sort of -ism that is degrading to another person.
Be Proactive. Use the 'Report' link on each comment to let us know of abusive posts.
Share with Us. We'd love to hear eyewitness accounts, the history behind an article.